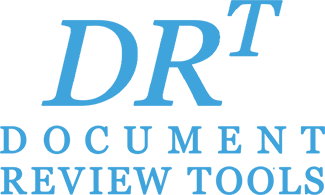
Performance
Our aim was to harness the expertise of the clinician by making NLP methodology accessible to the domain expert. In this approach, the clinician user operates the software to train NLP classifiers, run NLP queries, and abstract the data. This is a semi-automated review whereby the user reviews classified documents from within the software. For this reason, accuracy (a common metric for fully automated NLP systems) is no longer the most important performance metric. Users may choose to prioritize sensitivity over specificity, or vice versa, depending upon the prevalence and number of records to be reviewed for a particular project. A user/clinician may choose to perform a highly sensitive search by accepting a positive predictive value of 25%, e.g. review of 160 ED documents to identify 40 cases).
Project title | Records | Prevalence | Sensitivity | Positive predictive value |
---|---|---|---|---|
Burden of homelessness in ED | >460,000 | 0.1% | 97% (94-98%) | 72% (68-76%) |
Yield of CRP/ESR for fever of unknown origin | 6,700 | 6.0% | 94% (91-96%) | 34% (31-37%) |
Peri-orbital cellulitis who need emergent imaging | 670,076 | 0.2% | 91% (89-93%) | 19% (18-20%) |
Yield of Guaiac test for suspected intussusception | 178,112 | 0.7% | 88% (86-89%) | 72% (70-74%) |
Disparities in pain management among patients evaluated for acute appendicitis | 511,000 | 2% | 94.4 (92.5, 95.3%) | 41% (39.6, 42.8%) |
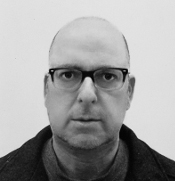
Contact:
Amir Kimia, MD
Solution Architect, co-founder
Associate Physician in Medicine, Boston Children's Hospital
Assistant Professor of Pediatrics, Harvard Medical School
Email: amir.kimia@childrens.harvard.edu
Phone: 617-355-6624